This week’s BEACON Researchers at Work blog post is by University of Texas at Austin research scientist Dennis Wylie.
I’ve always been intrigued by the combination of seemingly incongruous things. As a child I loved stories about strange chimerical creatures composed of one part this animal, two parts that, and so on — not just for the fantastic nature of the imagery but also because I couldn’t stop asking myself questions like “how does the goat head feel when the lion head eats a goat?” As an adult I’ve therefore found it particularly interesting to be working in the field of computational biology: the goals and methods of one discipline often seem bizarre from the perspective of another, but sometimes the contrast helps to bring out something new and unexpected.
Since January I’ve worked at the Center for Computational Biology and Bioinformatics (CCBB) at the University of Texas as part of the bioinformatics consulting group. We work with faculty members across several departments pursuing a large variety of different projects which in one way or another involve large-scale data sets and complex computational analyses, largely (though certainly not exclusively) based around next-generation sequencing (NGS). In the few months since I have joined the group, I have worked on projects applying computational approaches for NGS variant calling, methylation profiling via bisulfite sequencing, RNA-Seq differential expression analysis, RIP-Seq analysis, and metagenomics, among other methods.
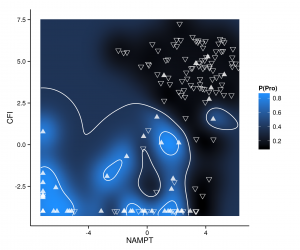
Probabilities of proneural vs. mesenchymal subtype (blue indicates higher probability of proneural, black of mesenchymal) predicted by toy SVM model designed to demonstrate overfitting and trained on RNA expression levels of two genes as measured by sequencing (GSE57872). Hollow inverted triangles are specimens which were assigned mesenchymal subtype according to Patel et al 2014 (Science 344: 1396-1401), while filled-in triangles represent specimens assigned to proneural subtype. Contours represent probability levels approximately equal to 50% or 100%; in each of the primarily mesenchymal and primarily proneural regions of the plot, a few samples of the opposite subtype create a “hole” region in which the prediction is flipped.
The project with which I have been most heavily involved here at UT is the ongoing study of the proteasome in Andreas Matouschek’s lab. While it has been well established for some time that the attachment of polyubiquitin chains to proteins targets them for degradation by the proteasome, some proteins are degraded with much less efficiency than others even when polyubiquitinated. Matouschek’s group has shown that the breakdown of polyubiquitinated proteins is accelerated by the presence of an unstructured region which can serve as an initiation site for the proteasome to begin the degradation process. Moreover, they have demonstrated through experiments varying the length and complexity of peptide tail sequences appended to fluorescent proteins that there are observable patterns in the types of peptides which serve as more or less effective initiation sites. The rich complexity of the space of relevant peptide structural features, combined with the increasingly large data sets the Matouschek lab is now generating, makes this a promising meeting ground for biophysical, computational, and statistical methodologies. In this context I am currently providing consultation applying algorithmic pattern recognition methods to help tease out in more detail which features determine proteasomal degradation efficiency.
At the CCBB I also have the opportunity to teach the methods I help apply in various research projects to students, postdocs, and anyone else who might be interested. At the end of every May we offer a slate of courses composing our “Summer School for Big Data in Biology,” for which this year I put together a course in machine learning methods for gene expression analysis (based both on my experience at UT and my prior work as a bioinformatician in industry developing molecular diagnostic tests). In planning the syllabus, assembling examples, teaching the techniques, and discussing the many potential applications with students, I had plenty of time to contemplate the interplay of ideas (and, more prosaically, of different jargons) from biology, mathematics, computer science, and many other fields. For example, the idea of model overfitting (e.g., see contour plot of overfit SVM classification model), whether done by human or by machine, lurks in the background of pretty much every scientific field (though not always known by that name).
It is my hope that in providing both research consultation and educational services at the CCBB we are assisting in the ongoing synthesis of scientific theory such that future generations will more easily appreciate the harmonies between disciplines. Combinations that today seem bizarre chimeras may one day be appreciated as natural fauna inhabiting the scientific landscape.
For more information about Dennis’ work, you can contact him at denniswylie at austin dot utexas dot edu.