This week’s Evolution 101 post is by MSU postdoc Bjørn Østman. Bjørn also blogs at Pleiotropy.
What is epistasis?
Epistasis is a measure of the strength of epistatic interactions. Epistatic interactions are non-additive interactions between alleles, loci, or mutations. That is, if the combined effect of a pair of mutations is not what we expect from their individual effects, we then say there is epistasis between those two mutations.
Two mutations that are both detrimental on their own can be beneficial when they occur together. An example of this is from Joe Thornton’s lab: the present function of reduced sensitivity to hormone in vertebrate glucocorticoid receptor is an example of this. Two mutations both reduced sensitivity and destabilized the newly duplicated gene shortly after its birth 450 million years ago. A third mutation – neutral without the first two mutations – buffered the destabilization, and allowed to gene to go fixation (Carroll et al., 2010).
Epistasis is mostly measured in terms of fitness, as the deviation from additivity, but in principle any trait-value can be used*. If mutation A increases fitness by 5% and B increases fitness by 10%, then we might expect that an organism with both mutations get a fitness increase of 1.05×1.10=1.155 or 15.5%. This would be the case if the two mutations do not interact, so that their effects on fitness are independent of each other. The deviation can be measured in various ways, but the proper way of doing it would be like this:
ε = log10[WAB × W0/ (WA × WB)],
where W0 is the fitness of the organisms with neither mutation. This is the best definition (!), because we assumed above that the effects of the mutations are to increase fitness by a fraction of the current fitness, rather than by adding a number. If mutations did increase fitness by an absolute number, we might measure epistasis as
ε = WAB + W0 – (WA + WB).
Both of these measures are then zero when there is no epistasis, and both can be extended to deal with more than two mutations interacting. When ε>0 we call it positive epistasis, and negative epistasis when ε<0 (Fig. 1).
So, if an organism with both mutations have a fitness of 1.20, then the amount of epistasis is ε = log10[1.20 / (1.05 × 1.10)] = 0.01660. If two deleterious mutations together have a beneficial effect, the sign of the joint effect is reversed, and this is called reciprocal sign epistasis (e.g., WA = 0.95, WB = 0.90, WAB = 1.20, giving ε = 0.1472). A trivial case of negative epistasis is when both mutations are independently neutral, but their joint effect is deleterious (e.g., WA = 1.0, WB = 1.0, WAB = 0.90, ε = -0.04576). I say this is a trivial case, because this type of interaction could be one where two genes carry out the same function, thereby exhibiting robustness by being redundant; the organisms then only suffers a fitness decrease when both genes are not working properly.

Fig. 1: Schematic illustration of epistasis. Two mutations A and B can interact epistatically in different ways with varying effects on fitness. The fitness of the wild-type is represented by the black baselines, and the heights of arrows represent the fitness after one mutation (WA or WB) and after both mutations (WAB). Green, positive epistasis, red, negative epistasis, black, no epistasis. In (a), two independently beneficial mutations may have their joint effect increased or diminished (WAB larger or smaller), while in (b) the independent effect of the two mutations is deleterious and beneficial, respectively, and the combined expected effect on fitness is deleterious. In (c), each mutation by itself is deleterious, but when they interact, the result can be reciprocal sign epistasis (green arrow). These sketches illustrate an additive model, where the sum of WA and WB is equal to WAB without epistasis. In our model, using the geometric mean this corresponds to taking the logarithms of the fitness. From Østman et al. (2012).
Epistasis is a feature of the genotype-phenotype map, and of genetic architecture. The genes that together are responsible for a trait (e.g., eyes, lungs, blood-clotting) are likely to interact and have non-zero epistasis. Many genes are also pleiotropic, i.e. part of gene-networks of more than one trait (Fig. 2), as they are expressed in different contexts (tissues, cell-types, in response to different environmental cues, etc.).
Fig 2: Epistatic modules. (A) Hypothetical genotype-phenotype map with three modules of groups of genes affecting three traits: eyes, lungs, and blood-clotting. The genes within each module interact epistatically, while some genes exhibit pleiotropy (black arrows). Not all pairs of genes affecting the same trait necessarily have a non-zero epistasis. (B) Human liver coexpression network and corresponding gene modules. The gene coexpression network consists of the top 12.5% most differentially expressed genes (5,012 expression traits). The colors of the nodes represent their module assignments. Each of the colors correspond to a trait, and most genes are only expressed in that trait, though some are expressed in more than one (pleiotropy), as indicated by lines signifying coexpression. From Friend (2010).
Why is epistasis important in evolution?
One reason why epistasis is so important in evolutionary biology is that it affects the fitness landscape. The structure of the fitness landscape in large part determines many important things in evolution, such as evolvability, robustness, repeatability, contingency, and speciation. If the environment dictates that on set of genes/loci has a particular combination of alleles that optimizes fitness, then without epistasis each gene can be optimized individually until the optimal combination is reached (i.e., there is one peak in the local fitness landscape, aka smooth landscape). Deterministically, the population will end up on the peak. However, if the genes/loci interact, then fitness values are modified, and the fitness landscape will no longer be smooth, but contain multiple local peaks with valleys in between. Evolution in such a rugged fitness landscape will not be predictable, and multiple outcomes are now possible. Because there are multiple peaks the population might get stuck on a local peak with lower fitness than the highest peak in the landscape. Another possibility is that more than one peak is climbed at the same time, and if such a situation can be sustained, it can lead to evolutionary branching and even speciation.
Another reason why epistasis is so important is that interactions between genes means that much more complex traits can be made. If genes did not interact
, then no trait would be affected by more than one gene (is this necessarily always true?). It is of course not possible to make a complex structure with only one kind of protein. Conversely, the more genes interact within a module, the more complex the trait can be, which in turn translates into higher fitness. With only a handful of genes available, only a simple eye can develop, while many genes together can make a more complex structure, which can increase the organism’s fitness. The fact that genes interact epistatically is why complex multicellular organisms with abundant cellular differentiation are possible at all.
How prevalent is epistasis?
Very. Basically, when people measure it, pretty much all pairs of mutations are epistatic. That’s hard to believe is true, and it probably isn’t. Measuring fitness is generally difficult; you have to measure the fitness of four organisms, and just a little bit of error will give ε different from zero. Therefore it is reasonable to attribute lots of non-zero measures below some limit to no epistasis. And then still, it turns out lots of pairs of mutations have significant epistasis between them. For example, Costanzo et al. (2010), using data from a genome-wide, quantitative analysis of genetic interactions in yeast, showed that even when including only high values of epistasis (|ε|>0.08), then a large fraction of gene pairs are epistatic (Fig. 3A). Or in Drosophila melanogaster, where 15 insertions in the genes involved in startle-induced locomotion show extensive genetic interactions (Fig. 3B)
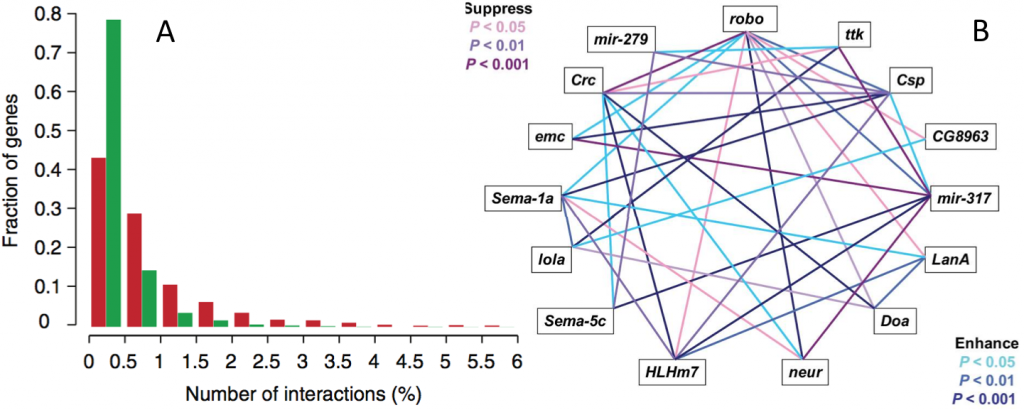
Fig. 3: Prevalance of epistasis. (A) The distribution of genetic interaction network degree for negative (red) and positive (green) interactions involving query genes. From Costanzo et al. (2010). (B) Epistatic interactions for startle-induced locomotion among 15 P[GT1] insertion lines in double heterozygous genotypes. From Yamamoto et al. (2008).
What is the current research focus?
Two major areas of research in evolution are adaptation and speciation. This has been so for a long time, and while we do know a lot about both, there is little doubt that this will not change in the foreseeable future. Adaptation is particularly affected by epistasis and pleiotropy, and it is an outstanding question to what extent adaptation is enhanced or mitigated by epistasis. Empirical data suggest that epistasis causes diminishing returns (e.g., Kahn et al, 2010), but this probably just means that the shape of fitness peaks are shallower the closer you get to the apex, which would just mean that the biggest returns on fitness comes with the first beneficial mutations (which are more likely to go to fixation in the first place). How much does epistasis affect evolvability? Fitness landscape ruggedness can limit a population’s ability to evolve, and ruggedness depends on the amount of epistasis among and within genes. But are these epistatic interactions set in stone, or are they malleable? In other words, how easy is it to create epistatic interactions, and once formed, can they be broken and allow for new advances in adaptation?
Speciation is also a much studied area of evolutionary biology, but the impact of genetic architecture is only recently coming into focus. Epistasis can cause Dobzhansky-Muller incompatibilities, which can lead to reproductive isolation (which is cool if your gold standard of speciation is the Biological Species Concept). But more generally, the epistastic nature of the genetic architecture causing multiple fitness peaks implies that evolutionary branching can occur. It remains an open question how much this is governed by epistasis, and particularly whether epistasis is a prerequisite for speciation of microbes.
* Not that I am thereby saying that fitness is just another trait. I hold the view that fitness – reproductive success – is a function of other traits, such that a network would point from genes to traits, and traits to fitness.
References
Carroll SM, Ortlund EA, and Thornton JW (2011). Mechanisms for the evolution of a derived function in the ancestral glucocorticoid receptor. PLoS Genetics, 7 (6) PMID: 21698144
Costanzo M, et al. (2010). The Genetic Landscape of a Cell Science, 327 DOI: 10.1126/science.1180823
Friend SH (2010). The need for precompetitive integrative bionetwork disease model building. Clinical pharmacology and therapeutics, 87 (5), 536-9 PMID: 20407459
Khan AI, Dinh DM, Schneider D, Lenski RE, and Cooper TF (2011). Negative epistasis between beneficial mutations in an evolving bacterial population. Science (New York, N.Y.), 332 (6034), 1193-6 PMID: 21636772
Yamamoto A, Zwarts L, Callaerts P, Norga K, Mackay TF, and Anholt RR (2008). Neurogenetic networks for startle-induced locomotion in Drosophila melanogaster. Proceedings of the National Academy of Sciences of the United States of America, 105 (34), 12393-8 PMID: 18713854
Østman B, Hintze A, and Adami C (2012). Impact of epistasis and pleiotropy on evolutionary adaptation. Proceedings of The Royal Society Biological sciences, 279 (1727), 247-56 PMID: 21697174
You can leave comments for Bjørn on his blog, where this is cross-posted: http://pleiotropy.fieldofscience.com/2012/09/epistasis-in-evolution.html