This week’s BEACON Researchers at Work blog post is by MSU graduate student Jory Schossau.
Have you ever played the game Rock, Paper, Scissors? Did you know you were mimicking the same sort of interactions that happen in communities of microbes? In a standard game we both pick Rock, Paper, or Scissors and reveal what we’ve picked. If I picked Rock, then you hope you picked Paper, because Paper wins over Rock. Scissors beats paper, and Rock beats Scissors. The game is interesting because no single play is best: there is always a way to win or lose no matter what you pick.
There are a few ways to make the game more interesting, and that is whether or not to play the game more than once, and if you play with the same person or not. With a repeated game, it gets even more interesting. That’s because you start experiencing the other player’s strategy, and changing your own. Perhaps your opponent usually picks Rock and you start catching on. But the interesting part is that they will experience the change in your own strategy and begin changing theirs as well. So who wins?
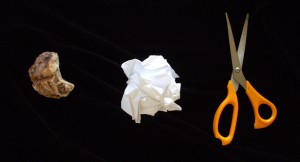
The traditional game Rock, Paper, Scissors uses imagery to help players remember the rules. Photo courtesy of Jesse Kruger.
I use this game for research, except I don’t just play one vs one, I make a thousand virtual creatures crowded together all play Rock, Paper, Scissors with their nearest several neighbors many times. One of the conditions I can change is the reward for winning using a particular play. Instead of rewarding one point for playing Rock, I could reward two points, which would make Rock a really valuable play, but possibly more predictable. Can you predict the outcome of this thousand-player community Rock, Paper, Scissors game?
Researchers like me use this game to study communities of organisms and predict how those population’s strategies will change over time given different conditions. Even behavior you might not think of as Rock, Paper, Scissors can be described and predicted using games like this. One organism we studied had the interesting behavior that it would sometimes explode, killing itself, but release a poison that kills its enemy or brethren if they aren’t immune. You might think this sort of behavior is self-defeating, and you are somewhat correct. To say it is really bad for the individuals who commits suicide is an understatement. But the nearby surviving immune members of the original community get a huge benefit from this self-destructive behavior because now they can eat the food left uneaten by the enemy. What a weird strategy! How does something like this come about?
In the biological scenario above, organisms don’t move around much, so they live and die in clusters within the larger community. Because genes are passed from parent to child then you can expect clusters of organisms to be closely related. Furthermore, those genes represent a strategy for life. This means a whole cluster of organisms can share the same genes, but some will be self-destructive and others not. It is the clusters of organisms as a whole that represent a strategy, whereas each organism is a single ‘play’ from that strategy and cannot change during its lifetime.
If genetics determines this self-destructive behavior, then clusters with the right amount of self-destructiveness benefit greatly from the killing power of the self-destructive’s poison which leaves extra food around so more offspring can be made. Those offspring, who may be more in number now than their ancestors, will have similar genetics to their ancestors. In contrast, clusters of organisms who develop bad genetics, such as never self-destructing, or always self-destructing, will decline in number over time because they don’t do as well or rarely produce offspring. In this way a strategy to sometimes play ‘self-destruct’ can become the dominant strategy in a community.
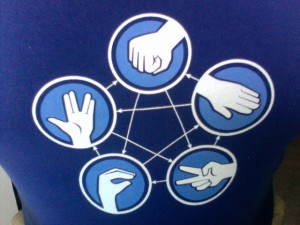
An extension of the classic game, known as Rock, Paper, Scissors, Lizard, Spock. Photo courtesy of Jose Silva.
To be able to see what would happen in different circumstances I used the Rock, Paper, Scissors game to approximate and simulate these biological interactions, but with evolution and a bit of genetics added to the normal game. While these games are simple, using a computer allows me to simulate thousands of games across thousands of generations and to track changes in the population genetics. The genetics is important because it determines which of three plays a new organism would become. That is, the strategy is how often will a new organism be Rock, Paper, or Scissors and the organism will play that for its entire lifetime. It is the genetics which determine what it will be when it is born. In my simulation the three plays correspond to Normal, Immune, and Immune with Self-Destruct. How often these plays occur in the community changes depending on a number of conditions, such as how costly it is to carry the genetic material for immunity, or the deadliness of the poison released by self-destructing. It is through models like these that help researchers understand and predict how natural communities of organisms change over time which is very useful if we depend on those organisms, or want to change those communities.
I haven’t always known I wanted to work with computers to help answer life’s persistent questions. At one point I was close to finishing school with a music degree. Serendipitously, my experience in a summer Research Experience for Undergraduates program let me explore my undiscovered interest for both computing and studying the complex and amazing relationships that make up life. Music is still a big part of my life and it finds a way into my research from time to time whether reviewing a paper about musical evolution, or finding new ways to measure the complexity of a composer’s style based on brain research. Who knows, maybe even musical game theory has a place in my academic future!
For more about Jory’s research, you can contact him at jory at msu do
t edu.