This week’s BEACON Researchers at Work blog post is by MSU graduate student Cory Kohn.
Skepticism. This is generally an important characteristic of scientists. Why would an attitude that is to be avoided in polite conversation act as a useful, even important, part of the scientific mindset? Good hypotheses will stand up to rigorous scrutiny. Those aspects of the explanations constructed to explain the world around us that do not stand up to scrutiny need to be re-evaluated. Skepticism is at the heart of both validation for good hypotheses and identification of those parts of science that need to be re-evaluated. Thus, being skeptical about what we think we know – and more importantly why we think we know it – is integral to advancing our understanding of the world around us. Skepticism is not about vicious personal attacks, but the key to assessment of one another’s results and methodologies so that we have a greater chance of formulating an accurate knowledge base about the world. Skepticism is my specialty – I test just how well scientific methods work as tools to understand the evolutionary history of organisms.
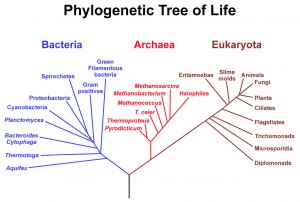
A phylogenetic hypothesis depicting the evolutionary relatedness within and among Bacteria, Archaea, and Eukaryota.
Specifically, my research involves testing the methodologies we use in phylogenetic inference. Phylogenies are the branching patterns that illustrate common descent. They depict the historical relationships between taxa by chronologically showing who is more closely related to whom. A phylogeny is by necessity a hypothesis – none of us actually witnessed the speciation events that gave rise to the diversity of life.
We construct our phylogenetic hypotheses by using the data we currently have available. Historically this data was primarily morphological, or perhaps behavioral; hypotheses about evolutionary relationships were the purview of taxonomic specialists who, through considerable experience, developed such a high degree of familiarity with their specimens that they could identify the key characteristics of organisms that indicate the historical pattern of relatedness. The problem with this methodology is the potential ambiguity of determining which characters indicate relatedness and not convergent or parallel evolution. For example, a non-evolutionary informative character would be the ability to fly. If we put significant weight in this character then we might hypothesize that flying birds, bats, and flies are more related to each other than they are to flightless birds, mammals, and insects. This highly simplistic example is illustrative of how problematic it can be to rely on characters that a researcher deems to be important. Cost, effort, and this issue of objective criteria for character selection is primarily why molecular sequence data, for example DNA, has increasingly become the data of choice over the past few decades. Such data can be used to hopefully construct an unbiased, more scientifically sound hypothesis regarding evolutionary relatedness.
With the molecular, computational, and statistically revolutions of the past few decades there is a plethora of phylogenetic methodologies and tools available for scientists to use in constructing their hypotheses regarding evolutionary relationships.
At first hearing it, you might be surprised to learn that none of these methods have been rigorously tested experimentally, but remember that phylogenetics involves the inference of historical patterns from existing (extant) data. Perhaps, regarding the validity of these tools, we aren’t being quite as skeptical as we probably should be.
That’s not to say these phylogenetic inference tools don’t work. They definitely have a sound theoretical basis and many have been tested using simulated sequence data. Yet simulated sequence data is only useful to test the range of conditions under which the simulation has been designed. Experimentally testing these tools would entail constructing a set of known descendant-ancestor relationships under biological conditions, using the resulting data to generate the hypothesized phylogeny, and comparing the results of the method to the known pattern of evolutionary relationships. Experimental tests have very rarely been done, for obvious reasons of labor and time. My research directly addresses this deficiency.
Experimentally validating phylogenetic reconstruction techniques is necessarily difficult when using biological organisms. One must observe evolution taking place, fully documenting the evolutionary branching pattern in order to compare the hypothesized phylogeny to historical reality. Feasibly, this can only be accomplished by using quickly evolving organisms such as viruses and perhaps bacteria.
I can generate even more information about the strengths and potential deficiencies of phylogenetic tools through using non-biological yet fully evolving populations. The artificial life platform Avida makes it relatively quick and easy to evolve a known evolutionary branching pattern. Further, I can know much more about my digitally evolving system than I otherwise could if I used biological organisms. I have a record of the genome for every digital organism in each of my populations. I can determine which mutations gave rise to phylogenetically informative or potentially misleading information, the evolutionary processes that gave rise to observed patterns, and these data allow me to directly understand how phylogenetic tools can generate inaccurate or misleading results.
Unlike simulations, an actually evolving system can be directly subjected to virtually all complexities found in biological reality. For example, while selection is for the most part disregarded in phylogenetic inference methodologies, I can evolve my populations under varying degrees of selective pressures to understand how selection influences the results of these methods. I can further manipulate genetic recombination, and the complexity of the known biological relationships by altering the number of taxa, amount of evolution between branching events, and degree of asymmetry in the branching structure.
An example of the true power of digital evolution is shown with selection. I can determine the exact fitness consequences of each mutation that eventually become fixed within a population. I can also determine how long each mutation takes to become fixed and when along the branching structure all of this occurs.
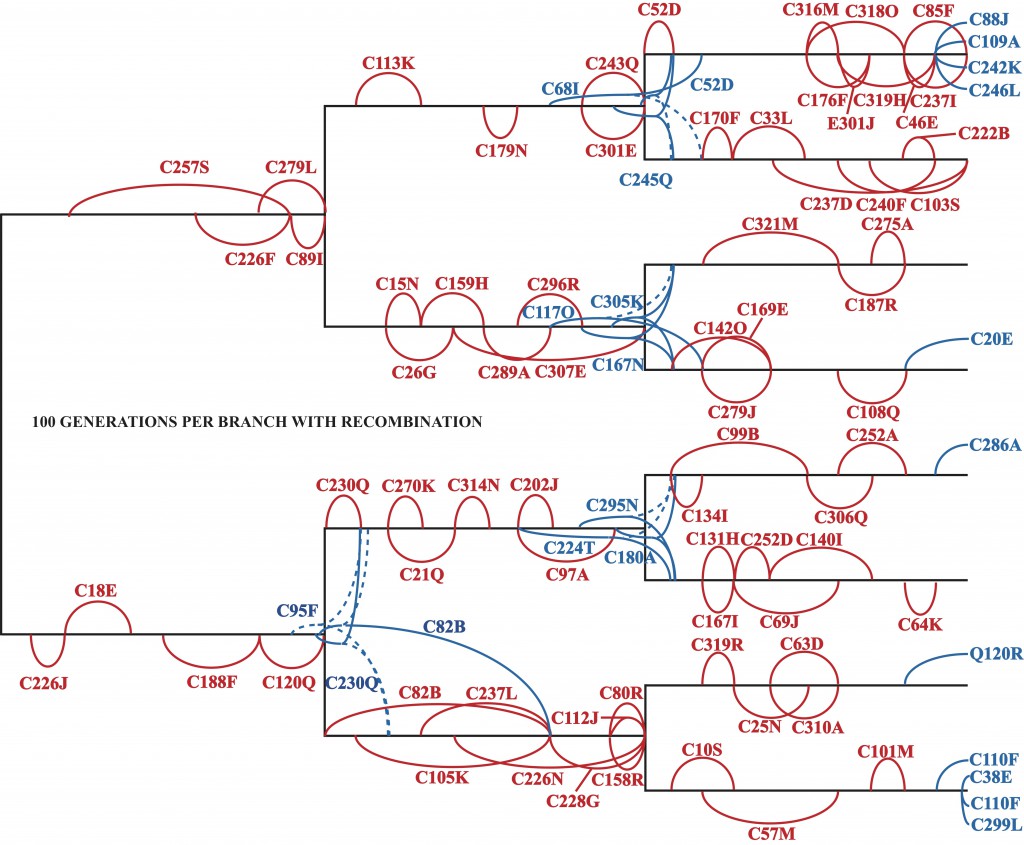
The known evolutionary relationships among eight Avida lineages evolved with recombination for 100 generations along each branch. Super-imposed along this phylogeny is the identity of every mutation that became fixed in the population, ie was eventually possessed by each member. In red are mutations that arose and became fixed along a single branch; in blue are those that became fixed on a subsequent branch, with a dashed line indicating a loss of the mutation in the sister population. For example, C257S (along the top left branch) denotes that the instruction C at genomic location 257 mutated to S around generation 20 and became fixed at generation 90.
In sum, digital evolution affords me the ability to precisely understand the range of conditions under which current phylogenetic methodologies are valid, and further, identify any potential shortcomings of these methods so that we can construct even better tools. Being skeptical has its benefits.
For more information about Cory’s work, you can contact him at kohncory at msu dot edu.