This week’s BEACON Researchers at Work blog post is by MSU graduate student Masoud Mirmomeni.
“Space Weather” hazards have achieved a great international scientific and public importance in recent years due to their catastrophic effects on modern technologies such as satellites and other distributed facilities. Today, space weather is a branch of science that will give new insights into the complex influences and effects of our violent Sun and other cosmic sources on interplanetary space, the Earth’s magnetosphere, ionosphere, and thermosphere that can influence the performance and reliability of space-borne and ground-based technological systems, and beyond that, on their endangering affects to life and health (Bothmer & Daglis, 2007; Moldwin, 2008). The Sun–Earth system is a complicated time varying system, ranging from magnetic field reconnection and accelerated solar wind as hot plasma to impact of charged particles on manmade electronic devices and biological systems.
The effects of our violent sun, as the main source of space weather disturbances, on our space environment ranging from producing faults in spacecraft operations to disruptions of distributed electrical power systems to the manufacturing of precision equipment have been well documented for more than 35 years (Kane, 2006). Space weather hazards on average cause annual losses of the order of more than $100 million (Maynard, 1990). The figure at right shows the known space weather effects on manmade technologies.
Considering the catastrophic effects of space weather on human technology, accurate predictions of space weather indexes seems to be an urge for modern society. During the past two decades, scientists have been working on this problem and have introduced different approaches to predict solar and geomagnetic activity indexes (Feynman and Gabriel, 2000; Vassiliadis, 2000).
In this research, we evolve Markov networks (MNs) (shown in figure below), which are probabilistic finite state machines (Edlund et al., 2011; Marstaller, Hintze, and Adami, 2013), to predict one of the famous “space weather” indexes in long-term: Sunspot number (SSN) (shown in graph below and are caused by intense magnetic activity, which inhibits convection by an effect comparable to the eddy current brake, forming areas of reduced surface temperature). These networks do not have the mentioned limiting assumptions on model structure and inputs. These networks choose the most informative inputs and the optimal structure through the course of evolution for a given problem; therefore, evolution helps us to solve input selection and structure system identification problem simultaneously.
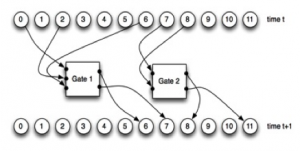
A Markov network with 12 nodes and two Probabilistic Logic Gates (PLGs). Once the nodes at time t pass binary information into the PLGs, the PLGs activate and update the states of the nodes at time t+1.
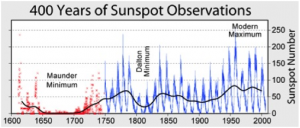
Sunspot number time series from 1600, showing the 11-year cycles of solar activity. Before 1750, the record is yearly and sporadic, after that we have monthly and daily data.
By using evolutionary algorithms, we are able to discover Markov networks that are able to predict SSN index accurately close to its theoretic prediction limit imposed by the chaotic nature of the signal. We evolved Markov networks and predict daily SSN one-step ahead for different years and states of solar activity to compare its performance with other well-established methods. We found that on average Markov network had the best performance (shown below).
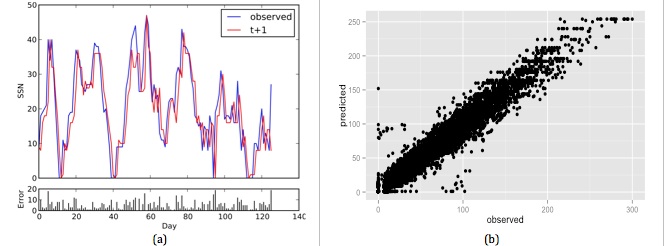
One step ahead prediction of daily sunspot number: (a) blue: actual sunspot number, red: one step ahead prediction. (b) one step ahead prediction vs. actual SSN time series.
Our ultimate goal in this project is to apply evolutionary algorithms to evolve Markov networks that are able to predict the solar and geomagnetic activity indexes near to their theoretic prediction limit imposed by the chaotic nature of space weather. By having an accurate prediction of these indexes, we can have an alarm system to avoid hazards of forthcoming geomagnetic storms on modern technologies. Moreover, we hope that by analyzing the structure of evolved networks, we are able to find time dependencies between lags of these indexes, which are difficult to capture with existing physical models.
References:
- M. Moldwin, An Introduction to Space Weather. Cambridge University Press, 2008.
- V. Bothmer and I. Daglis, Space Weather: Physics and Effects. Springer Praxis Books / Environmental Sciences, Praxis Publishing Limited, Chichester, 2007.
- I. A. Daglis, Space storms and space weather hazards, vol. 38. Springer, 2001.
- R. Kane, “The idea of space weather–a historical perspective,” Advances in Space Research, vol. 37, no. 6, pp. 1261–1264, 2006
- L. J. Lanzerotti, D. J. Thomson, and C. G. Maclennan, “Wireless at high altitudes environmental effects on space-based assets,” Bell Labs technical journal, vol. 2, no. 3, pp. 5–19, 1997.
- P.-N. Mayaud, Derivation, meaning, and use of geomagnetic indices, vol. 22. American Geophysical Union, 1980.
- J. Feynman and S. B. Gabriel, “On space weather consequences and predictions,” Journal of Geophysical Research: Space Physics, vol. 105, no. A5, pp. 10543–10564, 2000.
- D. Vassiliadis, “System identification, modeling, and prediction for space weather environments,” Plasma Science, IEEE Transactions on, vol. 28, pp. 1944–1955, Dec 2000.
- J. A. Edlund, N. Chaumont, A. Hintze, C. Koch, G. Tononi, and C. Adami, “Integrated informa- tion increases with fitness in the evolution of animats,” PLoS computational biology, vol. 7, no. 10, p. e1002236, 2011.
- L. Marstaller, A. Hintze, and C. Adami, “The evolution of representation in simple cognitive net- works,” Neural computation, vol. 25, no. 8, pp. 2079–2107, 2013.
For more information about Masoud’s work, you can contact him at mirmomen at msu dot edu.